New Go-playing trick defeats world-class Go AI—but loses to human amateurs
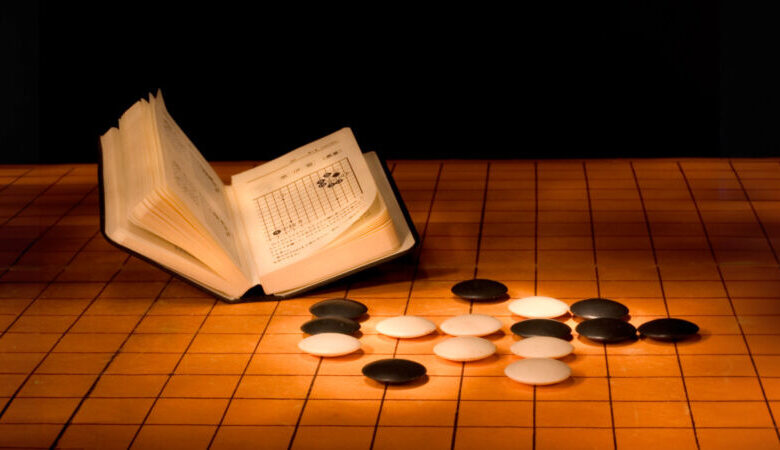
Enlarge / Go pieces and a rulebook on a Go board. (credit: Getty Images)
In the world of deep-learning AI, the ancient board game Go looms large. Until 2016, the best human Go player could still defeat the strongest Go-playing AI. That changed with DeepMind’s AlphaGo, which used deep-learning neural networks to teach itself the game at a level humans cannot match. More recently, KataGo has become popular as an open source Go-playing AI that can beat top-ranking human Go players.
Last week, a group of AI researchers published a paper outlining a method to defeat KataGo by using adversarial techniques that take advantage of KataGo’s blind spots. By playing unexpected moves outside of KataGo’s training set, a much weaker adversarial Go-playing program (that amateur humans can defeat) can trick KataGo into losing.
To wrap our minds around this achievement and its implications, we spoke to one of the paper’s co-authors, Adam Gleave, a Ph.D. candidate at UC Berkeley. Gleave (along with co-authors Tony Wang, Nora Belrose, Tom Tseng, Joseph Miller, Michael D. Dennis, Yawen Duan, Viktor Pogrebniak, Sergey Levine, and Stuart Russell) developed what AI researchers call an “adversarial policy.” In this case, the researchers’ policy uses a mixture of a neural network and a tree-search method (called Monte-Carlo Tree Search) to find Go moves.