Is Technology Only as Ethical As the People Wielding It?
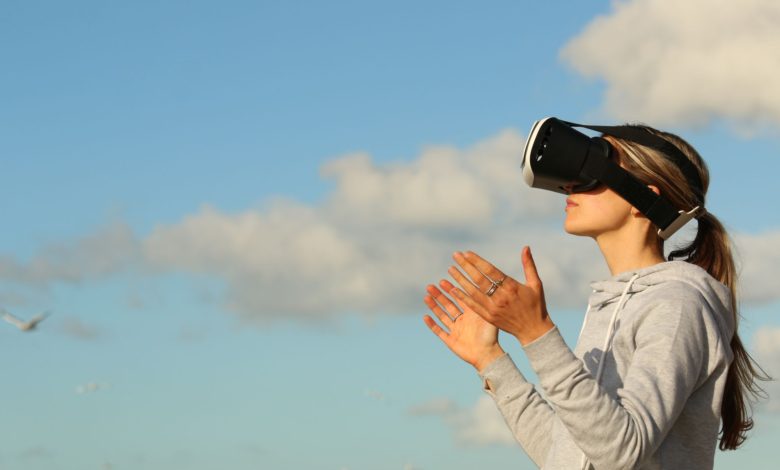
Technology is created by humans. Humans do not come in packages of “ethical” and “unethical.” Which is the primary reason why identifying ethical and unethical usage of technology is a rum business.
Since time immemorial, human progress has led to unprecedented discoveries, inventions and innovations. Yet, with the passage of time, the challenges, limitations and ethics of the progress have been less than perfect. Moreover, the situations or work conditions leading to that progress have been even more questionable.
One way of looking at the steep progress of technology in current times is to attempt to identify if technological progress is indeed correlated with the ethical barometer of the people using or creating it. Do the intentions of the creators of some technology define how ethical it is going to be, or can technology be made ethical on its own? Also, is ethics interwoven into certain technologies, and not in others, or is it simply the use cases of technologies which ascertain whether they are ethical usage or otherwise?
This then leads to the next question: so does technology require some sort of “policing” or regulation? Or should it be allowed freewheeling?
There is no single straight answer to these questions. But understanding the nature of certain technologies can suggest the route to the optimal solution(s). For illustrative purposes, a few examples of technological disruption have been explored here.
#1 Can Ethics be Interwoven into Technology?
When humans fail at something, the hope gets pegged on technology to show the way forward. Except that it is humans who create technology too. That defines the inherent challenge in interweaving ethics into technology: even with the best intentions, is it reasonably possible to make some tech innovation also ethical? There are scores of examples that can be considered, but AI is a “hot” topic of discussion of late, as far as its bias and usage goes. Can we hope that AI will solve a problem humans have trouble solving—removing bias in important decision making processes?
Can We Fix Biases in AI?
Artificial intelligence and machine learning are all pervading. They exist in our digital surroundings like the five elements. They are so inconspicuous, that we often do not realize that we are a part of some algorithm, knowingly or unknowingly. We also do not realize that we are, possibly, controlled by algorithms, and our lives are affected by algorithms.
So what happens if the algorithm has a ‘bias’? Bias means different things to different people. But in the context of decision making, Professor Mayson explains what ‘bias’ means:
“For some people, to say that a decision procedure is “biased” is to say that it is statistically unsound. A risk-assessment algorithm is racially biased in this sense if it systematically over- or understates the average risk of one racial group relative to another.”
Although this has been an unanswered question since the beginning, implementation of AI algorithms, especially in the judicial system, police systems and hiring, started receiving a deeper inquiry after the exposé by Pro Publica on “Machine Bias.” In the ongoing dialogue on eliminating racism, there has been an even bigger spotlight on the role of AI in perpetuating racism, especially in predictive policing, where the data is often not reflective of actual guilt of the candidates to begin with, and used in ways that are unaccounted for and opaque. In certain instances in UK, the predictive nature of AI skewed the decision of the police to make arrests: they arrested people as a self fulfilling prophecy. They made arrests because the algorithms said so, rather than actual need.
There are several things going on here. The most important aspect of machine learning is that it trains on data. It ‘predicts’ outcomes based on the input data. So if there was bias in the decision making process of past decisions, which make up the data, the ‘predicted outcome’ will simply carry forward that bias. As Prof Mayson explains,
“All prediction functions like a mirror. Its premise is that we can learn from the past because, absent intervention, the future will repeat it. Individual traits that correlated with crime commission in the past will correlate with crime commission in future. Predictive analysis, in effect, holds a mirror to the past.“

The second aspect is that machine learning algorithms do not predict the truth. They predict what is the most probable outcome. So an algorithm in predictive policing will only predict whether someone is likely to be arrested in the future or not, not whether they are actually guilty or not. If we feed ‘truthful’ data into the same algorithm, it will predict more truthful outcomes. Causality is not the biggest strength of ML. To assume a causal relationship because of the predictions is a folly.
The last, but perhaps most important aspect, is that human bias is irrational, and exists without even the knowledge of humans themselves, whereas AI has no inherent bias of its own (unless thus programmed). This is the most redeeming factor of AI. It is essentially memory-less outside the sphere of the training data, unlike humans. Professor Mullainathan explains this thus:
“We don’t just look at objective data; we also add our own internal biases. Study after study has demonstrated that when viewing a man and a woman doing a task at the same level of performance, people will make inferences about the woman they don’t make about the man. The mind just adds its own bias. The algorithms, while they may have other problems, tend not to add their own biases. They tend to reflect whatever is in the data.”
In a study on discrimination in AI, the authors explain that whereas it is practically impossible to analyze human bias, it is not impossible to do so with AI. This requires regulation over the algorithm and the training data. It requires storage and examination of the training data. Most importantly, it requires that the people who build it are conscientious of the perils of using wrong parameters in algorithms. Building trainer and screener algorithms to minimize discrimination is achievable, and greatly depends on regulation of humans who create and store the data and the people who build the ML models.
As Professor Mullainathan sums it up,
“The science is moving forward, and that means we can make the builders of these tools knowledgeable enough about bias and how to fix it, so that in 10 years what we’ll be left with is intention. It’s not going to be a technological problem; it’s going to be a sociological problem.”
Though it might look like this is uphill work, going by the news reports, there are already multiple projects in place which help achieve different measures of “fairness” in Machine Learning. Fairlearn is one such project which helps achieve fairness in Python. AI Fairness 360 Open Source Toolkit by IBM is another such package available for R and Python. It lists more than 70 metrics for measuring and achieving fairness.
With the right intentions, we have a trajectory to follow for achieving ethics or some measure of unbiased-ness in AI. Whether we achieve it or not, is a whole another question.
#2 Ethical Usage of Technology
The second way to look at the burgeoning technology is that some of the innovations could possibly be innocuous by themselves, but the use cases they are put to, affect people detrimentally or are tantamount to serious privacy violations. Some pertinent examples are discussed on the same.
Are All Use Cases of AI Ethical?
China has been at the forefront of using AI in numerous ways, which involve mining data of all sorts, including that of minors. In a research experiment, the Chinese authorities made children in a school wear AI powered devices that allows teachers and parents to monitor their concentration level as a live feed. This is then used to make the child focus on the class, if s/he is consistently not focusing as per the data. (As shown in the embedded video)
Assuming the school authorities have obtained consent of parents for mining this data—since the parents get a live feed of the activities of their kids—even so, monitoring every single activity of kids in a school, using AI devices, is questionable.
On its own, the AI device could be used in ways that are not interruptive to the learning process of children, but as of now, it does not leave room for kids to be playful or have fun in the classroom. A lot of children learn through interacting with others, or on their own, with toys. Smarter children often do not need to spend the same amount of time or the same type of effort to get the same learning outcomes. But making children wear devices that monitors their concentration level forces children to “concentrate” all the time, which may be less conducive to growth of the child overall. It is not uncommon for children to learn more through “play” rather than through traditional teaching. This could reduce the child to just a learning machine, rather than a growing human.
A similar surveillance system is slated to be installed in 43,000 schools in Russia. Ironically, it is called “Orwell.” This is a face recognition system, which will be able to mine data of and monitor children in every school. This could help in ensuring safety of children, but it could also be misused if the data is in the wrong hands.
Contact Tracing in the Time of COVID
When a contagious disease is on the spread, the top most challenge is to trace its spread, to find the “R0”, to test and quarantine the possibly infected people in time, thus contain its spread. This problem is solved by contact tracing better than any other means available to humanity at this point of time. Doing this task manually is not only unreliable, but not feasible when the numbers start swelling up. It is difficult—not to mention risky—for health officials to go knocking on the doors of potentially infected people, asking who all they have met.

Contact tracing apps help solve this problem by tracing the movements, symptoms, contact radius and other relevant metrics using GPS and bluetooth. South Korea effectively used such data to trace infected people and contain the spread. It also helps in dissemination of alerts and important information to people. Currently, there are more than 80 contact tracing apps available in 50 countries.
But for contact tracing apps to be really effective, they rely on adoption by all, or a sizable percentage of the population (atleast 60% as per some studies though that figure has been contested). If there are blind spots in the data, the predictions of “exposure risk” can be misleading. So the real challenge is whether it should be made optional and incentivized or mandatory by the government? Different countries have adopted different strategies. Singapore tried to give encouragements for adoption of their app.
But the most alarming aspect of contact tracing apps is that some countries do not even have a privacy policy in their apps! Some other apps have trackers from Google and Facebook in their code. All of this raises serious questions about who has access to the data and where it will be used, to what purposes. This stirs a lack of trust on the app, thus reducing its adoption by people. It also raises doubts about whether the apps are being used for the purpose stated or will they prove to be a liability a few years down the line? This is the classical problem of ethical use cases of tech innovation. The innovation itself could be life saving, but its usage must be limited for the stated purpose alone. To achieve this, a set of guidelines published by Nature help navigate the way forward in contact tracing. Decentralization of collected data is another system that reduces the privacy concerns, while at the same time, reducing the accuracy and corpus of data and control available to authorities.
#3 Ethics (or lack thereof) in Intention and Implementation of Technology
Sometimes, human intentions are the reason behind unethical innovations and implementation of new scientific/technological innovations. Crony capitalism, exploitation of masses, keeping users in the dark, causing deaths and diseases, all of these land us in a quagmire of innovations that take us several steps forward scientifically, but many giant leaps backward in terms of ethics.

Bayer & Their Legacy of Lawsuits
The supreme example of unethical innovation of technology and science that directly harms people is the Big pharma giant Bayer and their 45,000 law suits. These law suits are filed against Bayer for malfunctioning or adverse side effects of their medical devices, pharmaceuticals and herbicides and pesticides. Their products have caused conditions like unwanted pregnancy, fetal death, forced hysterectomy, autoimmune disorders, hair loss, life-threatening bleeding, and non-Hodgkin’s lymphoma, a form of blood Cancer (caused by Monsanto’s Roundup).
What’s worth noting is that many of these products have been around for decades, and their side effects or malfunctioning were discovered only much later. This clearly points to flaws in testing and approval of these products in the first place. So it is not a mere question of an innovation that went wrong, but many steps in its testing, approval and implementation that went terribly wrong, and was paid for with people’s lives. Worse still, though some of the products were recalled from the market, the weed killer Roundup is still around, and Bayer is investing further into its development. There isn’t even a conclusion on labelling their products with a warning.
This is not merely unethical, it is criminal.
So Where Do We Draw the Line?
Sometimes the glamour of growing technology is so high, that we forget that at the end of the day, it must have a purpose, and that purpose must be humane. Technological progress is meaningless if unnecessary suffering and human lives are spent in the process or as an outcome of that progress. How can that even be called progress?
So if we are to really trace the chain of ethics in tech, it would go from #3 to #2 to #1: Ensuring that the purpose of the innovation itself is ethical, then ensuring that it is used in ethical ways, and then ensuring that the technology itself does not include unethical treatment of any communities or people.

If all three are ensured, we can rightfully rejoice in the upward journey of ethical tech innovations.
References:
- Kleinberg, Jon and Ludwig, Jens and Mullainathan, Sendhil and Sunstein, Cass R., Discrimination in the Age of Algorithms (February 5, 2019). Available at http://dx.doi.org/10.2139/ssrn.3329669
- Skeem, Jennifer L. and Lowenkamp, Christopher, Using Algorithms to Address Trade-Offs Inherent in Predicting Recidivism (April 17, 2020). Skeem, J. & Lowenkamp, C., Using algorithms to address trade-offs inherent in predicting recidivism. Behavioral Sciences & the Law, Forthcoming, Available at SSRN: http://dx.doi.org/10.2139/ssrn.3578591
- Mayson, Sandra Gabriel, Bias In, Bias Out (September 28, 2018). 128 Yale Law Journal 2218 (2019),, University of Georgia School of Law Legal Studies Research Paper No. 2018-35, Available at SSRN: https://ssrn.com/abstract=3257004
- Larson, K., 2020. Contact Tracing Technologies: Methods And Trade-Offs – MIT Media Lab. [online] MIT Media Lab. Available at: https://www.media.mit.edu/publications/contact-tracing-technologies-methods-and-trade-offs [Accessed 25 July 2020].
- Morley, J., Cowls, J., Taddeo, M., & Floridi, L. (2020, June 4). Ethical guidelines for COVID-19 tracing apps. Nature, Vol. 582, pp. 29–31. https://doi.org/10.1038/d41586-020-01578-0
Cover image by Bradley Hook from Pexels